Big data requires big analysis, or should that be big mining? Read on to learn the difference
by Bethan Rees
Data analytics, according to our Professional Refresher module, is the "field of applying qualitative and quantitative techniques to analyse large amounts of raw data in order to draw conclusions and achieve gains for the organisation utilising the information".
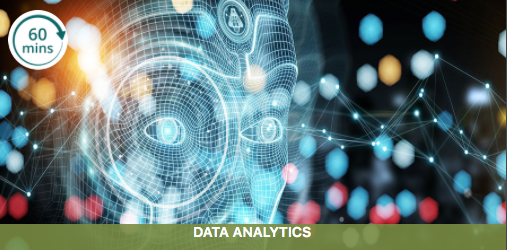
Data analytics uses sophisticated statistical and mathematical techniques to draw useful conclusions from the data sets generated by society. It can answer questions such as:
- How will our customer demographics change over the next ten years?
- How can we reduce our exposure to fraud?
- How are ethical investing trends impacting our business and the sector as a whole?
- What is a reasonable prediction for our sales figures for the next quarter?
- How can we increase our operational efficiency?
Here are five things worth knowing about data analytics, taken from our Professional Refresher.
1. Types of information
Big data "describes the gigantic amount of data we now create … that is almost impossible to manage and process using traditional business tools”. Within this field of data, information tends to be categorised in three groups.
Structured data – "organised to a specific format with predefined fields", such as daily stock prices sampled over ten years.
Unstructured data – no predefined structure or order, lacking "labels or other descriptive information", such as social media posts or images. It can be more complex to analyse.
Multi-structured data – combines structured and unstructured.
2. Types of data analysis
Descriptive – Aimed towards understanding the general characteristics of data, such as summary data, and including figures such as means, quantiles and correlations.
Diagnostic – Attempts to explain why something happened. An organisation may ask why sales in certain product lines increased while others fell over a time period; a theory such as a change in consumer preferences may be put forth, “then the analyst would search the existing data or seek additional inputs to see if these support that theory”.
Predictive – Attempts to predict the future. More complex than both descriptive and diagnostic, as it compares against behaviours or phenomena, which can be unpredictable. It can involve building a model created on the findings from descriptive or diagnostic analysis, "which will take a set of inputs and attempt to accurately predict some future event or state".
Prescriptive – Finding the best course of action based on all the information available.
Once you have analysis for forecasting, you can plan for actions with better outcomes.
3. Data mining
This is "a set of techniques or procedures used for the extraction of information from large data sets". It could involve searching for patterns or relationships among variables that aren't apparent. It uses tools and analysis techniques that can study large data sets that are too big for manual processing. Cluster analysis, for example, groups data together based on how closely associated it is.
"The difference between data mining and data analytics is a subtle one. Data mining attempts to discover hidden or unknown patterns in data. Data analytics, on the other hand, which includes forms of data analysis, is about testing hypotheses, undertaking focused research or constructing models in order to improve business processes and decision-making."
4. Data analytics and data protection
The EU General Data Protection Regulation (GDPR), as our Professional Refresher on GDPR explains, "addresses concerns around how personal data is used by public and private bodies" and "also accommodates a desire for people to control how their personal data is being used and provides greater transparency around its processing".
"Organisations conducting data analytics – and collecting data from the increasing range of available sources – must comply," says the data analytics module.
5. Pitfalls of assumptions
Incorrect assumptions about the data used for analysis and prediction can lead to disaster. Long-Term Capital Management (LTCM), a hedge fund in the 1990s, created “complex models to find small mispricings in financial markets”. These were exploited by using large amounts of leverage to hold large positions. LTCM thought the markets it had invested in had relatively stable correlations between assets, therefore buying a cheap asset and selling an expensive one was minimally risky.
However, market volatility in 1998 saw uncorrelated assets trading together, and LTCM began losing money. The situation was made worse by the leverage it had used. The impact of this could have been huge as the firm was a key player in the derivatives market, so a collapse would have affected the wider system and other trading counterparties. The US Federal Reserve had to step in and manage a recapitalisation. This "highlighted the risks of putting too much faith in particular historical relationships between similar assets, or trusting the stability of data used in models".