From GDPR considerations to data hygiene, there are operational issues to consider before implementing AI solutions
by Bethan Rees
A July 2021 CISI webinar, Operations forum: artificial intelligence implementation, provides insight into some of the behind-the-scenes issues and compliance elements to consider when looking to implement AI in a firm.
Frank Reardon, Chartered FCSI, chair of the CISI Operations Forum Committee and head of investment administration at JM Finn & Co, chairs the webinar. He is joined by three experts to discuss how they are approaching AI: John Pizzi, senior director of enterprise strategy for capital markets at FIS, Mohammed Gharbawi, senior fintech specialist at the Bank of England (BoE) and Blandine Arzur-Kean MCSI, independent compliance and regulatory consultant at Kean & Partners.
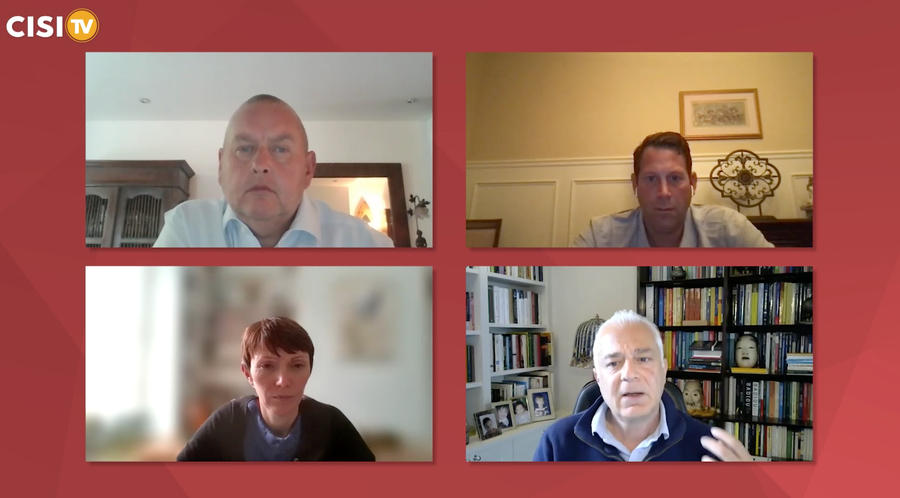
Pizzi kicks off the discussion by explaining where AI is heading. "Historically, people think about [AI] and think about robotic processes, how to make better automated activity, and obviously that’s a big part of financial services." However, over the past five years there’s been a "tremendous movement of data into the cloud" and a "proliferation of data", he says. Being able to comb through this data and understand it to find trends is where machine learning, a branch of AI that focuses on computer algorithms to automatically improve through experience, comes in. Machine learning can "comb through data at speeds that humans couldn’t, and find trends and key data points", says Pizzi.
The importance of machine learning and data science has increased as a result of the pandemic, says Gharbawi, referencing a report by the BoE. "[Banks] recognise that this is an area they need to be in", but this increase is not matched by increasing budgets, so banks may not be able to keep pace, he adds.
Preparing for AI
There are some elements to consider before implementing an AI solution into your processes. First is readiness, explains Pizzi. "You’ve got to have an organisational readiness around AI", which includes a "mind shift" towards data, as "the data you’re likely going to access is buried in legacy systems that aren’t monitored". The data needs to be accessible, so "you’ve got to reach back and spend some money and get that data into a data lake" [a repository, such as the cloud, that supports extremely large volumes of data, and can accept raw data], because "you’re not going to do AI out of the operating system itself".
"From a data protection perspective, it’s a minefield"
The second element needs to be an investment in people, he says, who you’ll need to figure out what to do with the data and, in turn, will need to create a "culture around what you’re going to do with the data". When people start analysing data in real time, a culture can emerge where ideas start to percolate and "data starts to become the currency for creating new ideas and … you’ve got the power of AI to be able to bring those ideas to light very quickly".
Another key element of preparing for AI is ensuring that your compliance and data protection teams are engaging with the project, and ensuring all stakeholders, for example, high-level senior management, understand AI and what it’s about, says Arzur-Kean. "If they don’t, it might lead to wrong decision-making," she says.
Data protection and data quality
AI is a data-driven process, and understanding what data to use can be difficult. "From a data protection perspective, it’s a minefield," says Azur-Kean. In the UK, there are two main pieces of legislation that are relevant. The Data Protection Act 2018 and the General Data Protection Regulation (GDPR), according to the Information Commissioner’s Office (ICO) guidance.
A June 2020 report by the European Parliament says that "AI is not explicitly mentioned in the GDPR, but many provisions in the GDPR are relevant to AI". It explains that there is a "tension between the traditional data protection principles", such as purpose limitation and treatment of sensitive data, and the full deployment of AI and big data. "However, there are ways to interpret, apply, and develop the data protection principles that are consistent with the beneficial uses of AI and big data," it says.
Some of the data used will need explicit consent, and you’ll need to review your data policy and contractual relationships to ensure you disclose the purposes for which the data is collected, says Azur-Kean. You must also understand what personal data is considered to be ‘sensitive’ and "what can be used on an anonymous basis to train your machine".
"Your models have to be sophisticated enough to look at the data and establish that it is bad"
Under Article 15 of the GDPR, a business using personal data for automated processing must be able to explain how the system makes decisions. An AI system "isn’t a big black box where stuff goes in and an answer comes out", says Pizzi. You have to be able to explain how the outcome was reached and why a decision was made. "That explainability factor is not something to gloss over, and if anybody’s doing AI and they don’t have a sound basis for the explainability, then they’re in big trouble," he says.
"Data cleanliness is an important part of any development effort," says Pizzi. The hardest part of AI, Pizzi says, is the data portion, rather than the AI portion. Having ‘dirty’ data or ‘bad’ data, such as duplicate, missing, invalid and inconsistent data sets, can lead to poor outcomes. Pizzi explains that the data is realistically not going to be clean when it comes in, "it's not going to be perfect". He says it's important to be able to handle bad data coming in and being filtered out. "Your models have to be sophisticated enough to look at the data and establish that it is bad."
Aside from data hygiene, Gharbawi says it's also important to know the lineage of your data – where the data comes from – in order to be able to use it properly. He says this specifically includes aggregated data – information that has been compiled into a summary or processed rather than raw data – which might be the case if you're looking at index data, for example. There may already be some "embedded biases" within the data proxies.
These are just some of the issues to consider ahead of implementing any AI solutions into your processes from an operations perspective. For more information, watch the full CISI webinar, here.